The complete guide to product analytics
The comprehensive guide for product analytics. Learn best practices and tactics used by top data-driven companies.
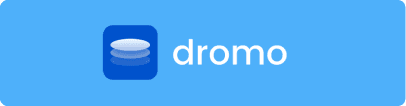

Authors of this article
Dave is a data guru and CEO of Dromo, a company that helps with data importing, normalization, and transformation. He's spent hundreds of hours setting up product analytics infrastructure.
Nick is the CEO of Signal, a product that creates data-powered territory plans for sales reps. He previously led a data engineering team at Okta before starting Signal.
Chase founded Flywheel after creating and launching Jira Work Management at Atlassian. He has executed product-led growth across multiple verticals and companies, ranging from SaaS to e-commerce.
Overview
Summary: Product analytics is the process of collecting, analyzing, and understanding data about users. It helps companies discover where users are finding value, surfaces areas for improvement, and makes it easier to optimize the user experience.
You’ve heard the term before. The words “product analytics” are thrown around by employees at every SaaS company and have a different meaning for everyone. If you’ve ever changed jobs, you’ll remember the pain of finding all the dashboards you need. Or, worse, they might not even exist and you’re the one responsible for building them from scratch.
Our goal is to get you fully up to speed on all things product analytics. Whether you’re just learning the basics or are delving deep into an implementation from zero, you’ll find the answers and resources you need in our guide to product analytics.
Here’s what we’ll cover in this guide:
What is product analytics
The importance of product analytics
Why product analytics is important for product-led growth
Best practices for product analytics
How to choose product analytics tools and software
Using product analytics to create tests and experiments
Using session recordings to watch user journeys
Real world examples and case studies of product analytics
Let’s dive in.
What is product analytics?
Product analytics is the process of collecting, analyzing, and understanding data about users. It helps you discover where users are finding value, highlights areas for improvement, and makes it easier to optimize user experiences. With product analytics, companies can make data-driven decisions about product features, retention, and conversion.
The first step towards product analytics is the process of tracking events. We’ll explore this in more detail later in the article, but the most important thing to know is that events are actions taken by a user. Tracking and storing these events allows you to measure product usage and understand patterns. Without events, there is no product analytics.
Now, it’s important to have a healthy relationship with product analytics. In past years, there has been a shift away from being “data driven” and instead towards being “data-informed”. This is largely due to cultures where decisions were stalled unless there was supporting data. This slows progress (imagine waiting for statistical significance on every decision) and keeps teams from their real goal — providing value for users.
And then there’s the other extreme. Making decisions based on gut instinct only seems to work when the PM has deep experience in the problem space. Executives are also able to shift the roadmap on a whim if the only rationale in opposition is a gut feeling. Product analytics can help companies make rational decisions and create consensus between opposing opinions. Think of product analytics as a guiding light that keeps you and your team on the right path.
So, while there’s always the risk of becoming too obsessed with analytics, not having visibility into product usage makes growing a software business much harder.
The importance of product analytics for software companies
The software world is competitive. Every year writing code and deploying features gets easier, while creating differentiated value for customers gets harder. Small teams can build large features quickly and iterate towards a great outcome. At least, when they’re well informed and understand what users want.
Product analytics drives this innovation. The needs of users change with the market, their company, their role, and as other software gets better. Talking with every user is practically impossible. Thus, product analytics comes in to provide a high-level overview of what your users are doing.
Here are a few more benefits.
Data-informed decision-making: Product analytics gives teams actionable insights into user behavior, allowing them to make informed decisions about product features, enhancements, and user experience. By using this data effectively, companies can optimize product performance, increase user satisfaction, and, ultimately, drive more revenue.
Improved user experience: Understanding how users interact with a product helps you continue delivering a seamless, useful experience. And that understanding needs to be in nearly real-time. Speed is key for identifying pain points, uncovering opportunities for improvement, and prioritizing product features based on data-driven insights.
Increased user engagement: By analyzing user behavior and engagement metrics, companies can identify trends, patterns, and areas for improvement. There’s also the ability to find which features create outsized value. Getting users to do what you want can be… frustrating to say the least. By finding the good and bad paths, users will have an overall better experience.
Why product analytics is important for product-led growth
Product-led growth (PLG) is a go-to-market strategy that makes the user experience the main lever for customer acquisition, expansion, and retention.The product itself becomes the core of marketing and sales funnels. You can read more about PLG basics in our article written in partnership with ClickUp and Atlassian here.
This huge focus on the product means understanding which features create value for users is the most important go-to-market input. In fact, product-led growth is probably the most data-driven go-to-market strategy. Should be, at least. PLG products who can’t deliver value within minutes will likely die away and be replaced by other, more obviously useful products.
This sounds a tad dramatic, but the truth is that sales teams are an unseen and steady crutch for marketing and growth teams. Account Executives and Sales Development Reps are incredibly effective at resolving user doubt on calls. No department sees more rejection than a sales team. In PLG, where sales talks mostly to enterprise leads, the “sales team” for small deals is your content, product experience, and pricing page. Coordinating all three to solve user doubt is hard. Product analytics makes it easier.
Of course, analytics applies to marketing, sales, and customer success, too. So, we also wrote guides for those disciplines.
Best practices for product analytics
Alright, so you understand why product analytics is important. You want to know how to actually do it. We'll walk through five steps of increasing sophistication to get you up to speed.
Step 01 (Beginner): Tracking user behavior
Step 02 (Beginner): Defining key adoption metrics
Step 03 (Beginner): Monitoring key metrics
Step 04 (Intermediate): Segmenting users into personas
Step 05 (Advanced): Just-in-time experiences
Step 01 (Beginner): Tracking user behavior
Product events, or user actions, take place across various platforms, including websites, software products, and marketing channels like email. Tracking these events is the first step towards product analytics.
Despite being the first step, most companies find tracking events to be hard. There’s a decision paralysis around naming conventions and which events to track. Our recommendations? Start with our list of 10 fundamental events. It’s a list of basic events that most B2B SaaS companies should be tracking.
The next level is to track specific events that are relevant to your specific product. The easiest way to do this is to use a tool that utilizes auto-track capabilities. Flywheel, Pendo, and Heap are all examples. Auto-track comes from a small code snippet that automatically tracks every user interaction where installed. This is a fantastic way to start learning about your users immediately. The downside of this approach — your data will be less formulaic and harder to aggregate at scale. Most companies start with auto-track and move towards manual tracking over time.
Examples of auto-track events look like this:
“Clicked button with text Sign Up”: Occurs when a user clicks on a button that has the text “Sign Up”. Straightforward.
“Played video”: Occurs when a user plays a video. Also obvious.
“Clicked p with text Flywheel EA • See how it works”: Occurs when someone clicks a specific element on our marketing website. Still descriptive, but if this button changes then the event will no longer fire in the same way. This highlights some of the limitations of auto-track.
If you’re a larger company or have access to engineering resources, you can opt to manually track events. Some products, like Flywheel and PostHog, support both paths. Manually tracking events requires an engineer to write code that triggers events when actions are taken. Each event must be given a name, ideally a descriptive one, and is set up individually.
The Segment B2B SaaS spec is a collections of recommended, manually tracked events. Here are some other examples:
"Page View" event: Occurs when a user visits a specific web page on your site. This helps you understand which pages are generating the most traffic. Most tracking snippets fire these events without requiring any engineering effort.
"Sign Up Button Clicked" event: Triggered when a user clicks on the sign-up button. This can help you identify the effectiveness of your call-to-action (CTA) and registration process.
"User Invited" event: Takes place when a user invites a team member to use your software or service. This event can give you insight into your product's vitality.
If you decide to track events manually, use a consistent naming convention. It should be a clear and consistent naming structure for your events. This will make it easier for team members to analyze the data and understand the metrics.
Step 02 (Beginner): Defining key adoption metrics
Now that you’re tracking basic events, it’s time to choose which ones that are most important. This will help you cut through the noise and focus on the user actions that truly matter. For a deeper look, check out our article on key product metrics for a full list of 39 to choose from, as well as how to decide which are most relevant.
To start, though, you’ll want to establish clear goals and metrics for product adoption, such as activation rate, feature adoption, and time-to-value. Look into these user journeys and decide which events correlate with success. Regularly monitor these metrics to evaluate the success of your adoption efforts and inform future improvements.
For example, Facebook is famous for tracking the number of friend requests sent by new users within a given time frame. Similarly, Jira keeps a close eye on the number of new signups that create 3 or more tasks in a new project. These are both user actions that occur relatively long after the initial signup event — but signal higher activation.
Pro tip: Expect major drop-off in your funnel. We’ve talked with hugely successful PLG companies that lose more than 60% of signups before they even get into the product. The culprit? A required email click to validate they're a real user. The volume of users that hit your key milestones may be very low.
Step 03 (Intermediate): Monitoring key metrics
This is where we finally get into what most people view as product analytics. Simply tracking and storing events won’t be very consumable or useful at scale, so most companies opt to use software for visualizing and querying the raw event data.
There are a few different approaches to monitoring metrics:
Manual queries
Periodic presentations
Product analytics dashboards:
Most companies decide to have some level of product analytics dashboard set up. We have an extensive set of dashboard best practices since the topic is large. Without going too in-depth, it’s normal to have a singular dashboard that contains your most important metrics, used alongside supplementary dashboards or charts for less critical metrics. Tools like Flywheel will build dashboards for you, or tools like Amplitude and Mixpanel allow for designing niche and in-depth dashboards.
Manual queries
Just like it sounds, these are queries that you run yourself. If you know SQL or other querying languages, you can directly access the raw data from your database or data warehouse and find answers to questions as they come up. Generally, there is a set list of saved queries that are run whenever you want the most recent update on a metric.
Periodic presentations
It's a bit of a stretch to call this a solution, but nonetheless it's common for executives to request presentations from data analysts. These presentations are generally held at a set cadence and go over key metrics, recent changes, and notable trends. The benefit of presentations is the ability to ask follow up questions and dive deeper into interesting insights. The negative is that you’ll often just get updates on the same metrics every time. Having a readily consumable dashboard replaces the need for this type of update and allows executives to spend more time diving deeply into anomalies.
Step 04 (Intermediate): Segmenting users into personas
Now that you’re tracking events and monitoring key metrics, it’s time to level up. Specifically, by tracking user activity based on the types of users they are. Segmentation is the key to optimizing your product and go-to-market strategy.
You’re likely familiar with the concept of an ICP, or ideal customer profile. Find software that helps you build out granular customer segments, then analyze the adoption patterns of those users. You’ll often find that the feature adoption changes from persona to persona, helping you build accompanying content or in-product help guides that can be tailored to that specific audience.
This level of segmentation can also help companies decide which users are important to include in feature analysis. For example, you may have a product that targets Product Managers. Once you've segmented by personas, you might find Content Marketers rarely adopt your product and are lowering your overall activation rate. Try filtering out that segment and re-analyzing your product activation metrics according to your core audience.
Most product analytics tools allow you to build user segments in some fashion. The real difference comes down to ease of creation. We've found that most companies don't take advantage of user segmentation features due to how confusing it is in legacy tooling.
Step 05 (Advanced): Just-in-time experiences
The last stage, and the most advanced, is delivering messaging in your product to users based on:
The customer segment they belong to
Previous engagement with your product
Overall company engagement with your product
This ladders into ABM tactics. You’ll no longer send irrelevant messages to users if you can avoid sending information about features they’ve already used. Additionally, if a content marketer hasn’t used a specific feature, but they also probably shouldn’t, you can reduce message spam.
These messages can be delivered in many formats. Tooltips, modals, pop ups, and messages through platforms such as Intercom and Drift are common examples.
Let’s go back to Atlassian. Jira is most often used by engineers and product managers. However, many marketers log into Jira track which features will be delivered shortly. This helps marketers build out content according to a product launch plan. Once you understand the behavior and rationale of these marketers, you can avoid messaging them about new backlog grooming features. Instead, Atlassian could inform these marketers about alerting functionality to help them stay on top of upcoming releases, automatically.
How to choose the right product analytics tools and software
There is a vast market of tools that surround the product analytics space. This article will touch on the three major tooling categories and what they’re used for.
Software for tracking product events
Product event tracking software captures and records events on your web surfaces, such as clicks, page views, form submissions, and feature usage. This data is invaluable for understanding user behavior and informing product analytics insights.
The output of this data is what to consider when making a choice. There are subcategories for event tracking, as shown below:
Customer Data Platforms (CDPs): A CDP will track and store your events, but they excel at sending that data to other software. You can think of a CDP as a middleman for your data. Segment and mParticle are two of the best examples.
Analytics visualization software: This category will take the tracked data and allow you to visualize it. Occasionally, this data can also be exported to other tools. However, the data will almost always be sent in its raw format. A few visualization tools also have event tracking code snippets, such as Flywheel, Amplitude, and Mixpanel. These snippets are sometimes referred to as SDKs.
ABM analytics software: These are tools that can automatically associate events with people and the companies they work for. Marketing motions focused on companies are called Account Based Marketing (ABM) campaigns. It’s a newer space with fewer options. Flywheel, Dreamdata, and Variance offer these capabilities for use cases.
Session recording software: While this is slightly different from the other categories, session recording tools track user events and turn them into videos. Flywheel, LogRocket, and FullStory are examples. These videos can be used to understand user journeys (Flywheel), or are optimized for discovering bugs in your software (LogRocket / Fullstory).
Ultimately, decide what you want to accomplish with your data and choose an event tracking software that provides that solution. One last consideration is to figure out which team members will want to see the analytics insights. The more people that will want to see the data, the more you should avoid software that prices per user.
Software for visualizing product analytics
Visualization software turns raw data into visual formats, such as charts, graphs, and dashboards. These visuals can help you quickly identify trends, patterns, and anomalies in your data. When evaluating visualization software for product analytics, consider these factors:
Granularity: Some tools excel at showing granular patterns per user, whereas other software gives great high-level overviews. No software does both excellently, so making a decision here will narrow down your choices. Flywheel is great for granular user and account insights, and Tableau is great for high-level overviews. Tools like Amplitude and Mixpanel are somewhere in the middle.
Ease of setup: The more complex your data model, the more likely you need a tool that takes weeks to configure. This will involve data analytics teams and engineers. If you’re starting out smaller, other software is considered “plug-and-play” and can start giving you insights in minutes.
Descriptive vs Prescriptive: Tools like Tableau became famous for allowing users to create visualizations to answer any data question. This is what we would call a “descriptive” product and outputs according to what you input. Flywheel uses a ‘prescriptive’ approach, where connecting a data source is the only setup configuration step. Flywheel will automatically give you insights and answers, but is more limited in the answers it can provide as a result. Align on your company’s preference before evaluating software, or give both a test run before deciding.
Software for surfacing hidden product insights
Some software helps you derive actionable insights from your product analytics data by identifying trends, patterns, and anomalies that aren’t obvious from raw data or visualizations. These tools use advanced algorithms and machine learning techniques to surface insights and recommendations.
This is a newer product category that has accelerated recently alongside the rise of GPT-4 and other large language models (LLMs). The benefit is finding insights you may otherwise miss. The downside is that the insights might be too granular. Discovering that mobile users from Country B adopt Feature X at a higher rate might not change your strategy or decisions. Or, maybe it will. Newcomers to this space include Fuzy.ai and Getloops.
Using product analytics to A/B test and experiment
The advanced use case of product analytics is to actually make changes from insights. These should affect the user experience. One of the best ways to accomplish this is through A/B testing and experimentation. There are many web surfaces that lend themselves to experimentation:
Marketing content and copy
Features and feature gating
Email content and automated email campaigns
Onboarding flows for new users
You’ll find that the best practices for experimentation are oddly similar to product analytics itself. Here are some of those best practices:
Define experiment goals and metrics: Establish clear goals and metrics for each experiment, such as user engagement, retention, and revenue generation. Experiments without clear goals are usually a waste of time.
Segment your users: Identify distinct user segments, such as power users, at-risk users, and new users. Or, group them by company industry and user title. Tailor your experimentation strategies to address the unique needs and preferences of each segment.
Test and iterate: Use software to enact different experiment designs, such as A/B testing, multivariate testing, and split testing. Try to have more than one test running at a time to avoid narrowing your focus. Also, occasionally test unconventional ideas. We routinely see “bad” ideas outperform preferred ideas.
Measure impact: Complete the experimentation loop by using your product analytics to measure the impact of your tests and experiments. Rinse and repeat.
Choosing an experimentation platform
The biggest factor in choosing an experimentation platform is to figure out who will be running the experiments. If it’s the engineering team or a growth team that can code, there are many feature flag-based experiment platforms. Some of these include:
Eppo
Amplitude
PostHog
Optimizely
Alternatively, if your marketing team wants to run experiments, you’ll likely need to choose a platform with no-code experimentation capabilities. The most popular of these is Google Optimize. Unfortunately, Google Optimize will no longer be available after September 2023. In response, multiple companies are filling the void. Some of these are:
Mutiny
VWO Testing
CrazyEgg
Flywheel
The key difference between no-code and feature-flag testing is where the experiments can be run. Most of the no-code experimentation platforms are intended to run experiments on websites. Platforms built on feature flags are better suited for product experimentation.
Many larger companies have a centralized experimentation team that is in charge of deploying experiments for all teams. We’ve found this to be less effective. This approach usually ends up with an overwhelmed experimentation team, which leads to descoped and limited experiments. Ideally, experiments should be run quickly, frequently, and without excessive time spent thinking. Thus, we recommend that product teams run point on product experiments and marketing teams own marketing experiments.
Using session recordings to watch user journeys
So now you’re tracking, visualizing, and experimenting. That’s a lot of data and systems. One way to make sense of them all is through session recordings.
Session recordings are an essential part of product analytics. They provide a visual representation of how users navigate through your product, helping you see what works well and where improvements need to be made. By watching user journeys in the form of video, you can identify common patterns or bottlenecks. You can also use them to watch the differences created by experiments.
We recommend using a tool that can record sessions across different domains. This allows you to watch a user visit your website, sign into your product, view your documentation, and take other circuitous journeys. Limiting the scope of session recordings to just your product means you’re missing out on most of the user journey. Particularly in product-led growth, the product is just a single touchpoint for most customers.
Session recordings have the ability to humanize your users and make them seem less like numbers. Building empathy for users often leads to different product prioritization decisions. An example learned from building Flywheel is the process of integrating 3rd party software. We’ve worked hard to make sure every integration takes less than three clicks. However, we occasionally see edge cases where a user gets confused in a way that we never expected. Viewing an event timeline of the same scenario doesn’t give the same clarity as watching the user attempt to integrate a data source in real time.
Identifying user pain points
One of the primary goals of using session recordings is to identify user pain points. These are areas where users encounter difficulty, confusion, or frustration while interacting with your product. By pinpointing these areas, you can prioritize and address them, leading to an improved user experience. This can result in increased customer satisfaction, reduced churn, and ultimately, higher revenue.
Benefiting from session recording insights
To get the most out of session recordings, it's crucial to analyze them systematically rather than randomly. For example, Flywheel highlights session recordings that contain key activation milestones, new signups, and recordings that involve important features. It's easy to get overwhelmed with the number of session recordings. Watching with specificity over simply viewing every user action will deliver insights more quickly. Alternatively, engineers might choose to only watch session recordings where errors occur.
Choose a session recording tool that has advanced filtering capabilities based on events within the session. A bonus is the ability to filter on user personas. This way you can quickly figure out how to improve retention.
Case studies: Successful product analytics implementation
That’s the full toolkit for product analytics. As we mentioned, there are follow-up articles on most of these topics where you can dive deeper. For now, though, it’s time to see how product analytics can actively change how teams work.
Case Study 1: Improving user onboarding with analytics
A SaaS company specializing in project management software used product analytics to identify areas where users were struggling during the onboarding process. By analyzing user session recordings and tracking key product metrics, the company discovered that a significant percentage of users were abandoning the onboarding process before completing the first crucial steps.
Armed with this insight, the company redesigned its onboarding process, providing clearer instructions, tooltips, and in-app guidance to help users quickly understand and navigate the platform. As a result, user engagement and retention rates increased, leading to higher customer satisfaction and revenue growth.
Case Study 2: Optimizing user experiences with product analytics
An e-commerce company used product analytics tools to track and analyze user behavior on their website. By monitoring metrics such as bounce rate, page load time, and conversion rate, the company identified several areas of their website that were underperforming.
To address these issues, the company implemented changes, including optimizing images for faster load times, simplifying the checkout process, and making their website mobile-responsive. These improvements led to a better user experience, increased conversions, and higher revenue.
Case Study 3: Enhancing customer support with product analytics
A B2B software company used product analytics to improve its customer support experience. By analyzing product metrics and user feedback, the company identified common pain points and areas where users needed additional assistance.
To address these needs, the company created targeted in-app support materials, including video tutorials and FAQ pages. They also used product analytics to track the effectiveness of these support resources, making ongoing improvements based on user engagement data. As a result, the company saw a significant reduction in support requests and an increase in customer satisfaction.
Fin
At the end of the day, product analytics is a powerful tool for businesses looking to build better user experiences. It can be hard to set up, hard to create the right visualizations, and hard to get teams aligned on what the results mean. But as we like to say, taking any step is progress. It’s easier to improve existing systems than to set them up from scratch. No matter where you are on the journey, we hope this article helps you take that next step. Cheers!
Published on
Apr 26, 2023
Next up:
Table of contents